Beyond Solr: Scale search across years of event data
Every company wants to guarantee uptime and positive experiences for its customers. Behind the scenes, in increasingly complex IT environments, this means giving operations teams greater visibility into their systems — stretching the window of insight from hours or days to months and even multiple years. After all, how can IT leaders drive effective operations today if they don’t have the full-scale visibility needed to align IT metrics with business results?
Expanding the window of visibility has clear benefits in terms of identifying emerging problems anywhere in the environment, minimizing security risks, and surfacing opportunities for innovation. Yet it also has costs. From an IT operations standpoint, time is data: The further you want to see, the more data you have to collect and analyze. It is an enormous challenge to build a system that can ingest many terabytes of event data per day while maintaining years of data, all indexed and ready for search and analysis.
These extreme scale requirements, combined with the time-oriented nature of event data, led us at Rocana to build an indexing and search system that supports ever-growing mountains of operations data — for which general-purpose search engines are ill-suited. As a result, Rocana Search has proven to significantly outperform solutions such as Apache Solr in data ingestion. We achieved this without restricting the volume of online and searchable data, with a solution that balances responsively and scales horizontally via dynamic partitioning.
The need for a new approach
When your mission is to enable petabyte-level visibility across years of operational data, you face three primary scalability challenges:
- Data ingestion performance: As the number of data sources monitored by IT operations teams grows, can the system continue to pull in data, index it immediately, store it indefinitely, and categorize it for faceting?
- Volume of searchable data that can be maintained: Can the system keep all data indexed as the volume approaches petabyte scale, without pruning data at the cost of losing historical analysis?
- Query speed: Can the index perform more complex queries without killing performance?
The major open source contenders in this search space are Apache Solr and Elasticsearch, which both use Lucene under the covers. We initially looked very closely at these products as potential foundations on which to build the first version of Rocana Ops. While Elasticsearch has many features that are relevant to our needs, potential data loss has significant implications for our use cases, so we decided to build the first version of Rocana Ops on Solr.
Solr’s scaling method is to shard the index, which splits the various Lucene indexes into a fixed number of separate chunks. Solr then spreads them out across a cluster of machines, providing parallel and faster ingest performance. At lower data rates and short data retention periods, Solr’s sharding model works. We successfully demonstrated this in production environments with limited data retention requirements. But the Lucene indexes still grow larger over time, presenting persistent scalability challenges and prompting us to rethink the best approach to search in this context.
Compare partitioning models
Like Elasticsearch and Solr, Rocana Search is a distributed search engine built on top of Lucene. The Rocana Search sharding model is significantly different from Elasticsearch and Solr. It creates new Lucene indexes dynamically over time, enabling customers to retain years of indexed data on disk and have it immediately accessible for query, while keeping each Lucene index small enough to maintain low-latency query times.
Why didn’t the Solr and Elasticsearch sharding models work for us? Both solutions have a fixed sharding model, where you specify the number of shards at the time the collection is created.
With Elasticsearch, changing the number of shards requires you to create a new collection and re-index all of your data. With Solr, there are two ways to grow the number of shards for a pre-existing collection: splitting shards and adding new shards. Which method you use depends on how you route documents to shards. Solr has two routing methods, compositeId (default) and implicit. With either method, large enterprise production environments will eventually hit practical limits for the number of shards in a single index. In our experience, that limit is somewhere between 600 and 1,000 shards per Solr collection.
Before the development of Rocana Search, Rocana Ops used Solr with implicit document routing. While this made it difficult to add shards to an existing index, it allowed us to build a time-based semantic partitioning layer on top of Solr shards, giving us additional scalability on query, as we didn’t have to route every query to all shards.
In production environments, our customers are ingesting billions of events per day, so ingest performance matters. Unfortunately, fixed shard models and very large daily data volumes do not mix well. Eventually you will have too much data in each shard, causing ingest and query to slow dramatically. You’re then left choosing between two bad options:
- Create more shards and re-index all data into them (as described above).
- Periodically prune data out of the existing shards, which requires deleting data permanently or putting it into “cold” storage, where it is no longer readily accessible for search.
Unfortunately, neither option suited our needs.
The advantages of dynamic sharding
Data coming into Rocana Ops is time-based, which allowed us to create a dynamic sharding model for Rocana Search. In the simplest terms, you can specify that a new shard be created every day on each cluster node: at 100 nodes, that’s 100 new shards every day. If your time partitions are configured appropriately, the dynamic sharding model allows the system to scale over time to retain as much data as you want to keep, while still achieving high rates of data ingest and ultra-low-latency queries. What allows us to utilize this strategy is a two-part sharding model:
- We create new shards over time (typically every day), which we call partitions.
- We slice each of those daily partitions into smaller pieces, and these slices correspond to actual Lucene directories.
Each node on the cluster will add data to a small number of slices, dividing the work of processing all the messages for a given day across an arbitrary number of nodes as shown in Figure 1.
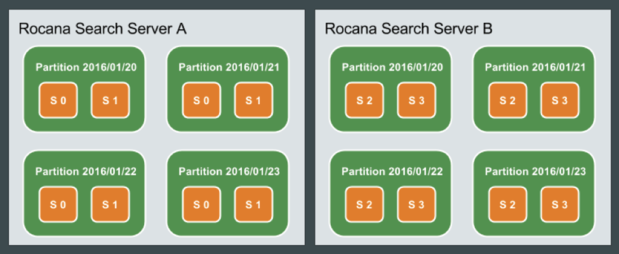
Figure 1: Partitions and slices on Rocana Search servers. In this small example, two Rocana Search servers, with two slices (S) per node, have data spanning four time partitions. The number of partitions will grow dynamically as new data comes in.
Each event coming to Rocana Ops has a timestamp. For example, if the data comes from a syslog stream, we use the timestamp on the syslog record, and we route each event to the appropriate time partition based on that timestamp. All queries in Rocana Search are required to define a time range — any given window of time where an item of interest happened. When a query arrives, it will be parsed to determine which of the time partitions on the Rocana Search system are in scope. Rocana Search will then only search that subset.
Ingest performance
The difference in ingestion performance between Solr and Rocana Search is striking. In controlled tests with a small cluster, Rocana Search’s initial performance has proved significantly better — as much as two times — than Solr, and the performance gap grows significantly over time as the systems ingest more data. At the end of these tests, Rocana Search performs in the range of five to six times faster than Solr.
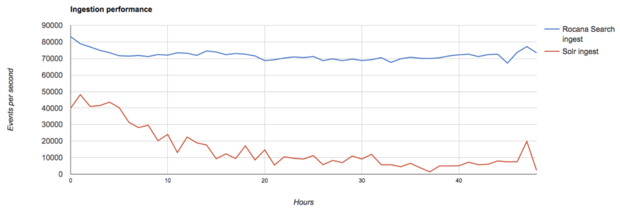
Figure 2: Comparing data ingestion speed of Rocana Search versus Solr over a 48-hour period on the same four-DataNode Hadoop (HDFS) cluster. Rocana Search is able to ingest more than 12.5 billion events, versus 2.4 billion for Solr.
Event size and cardinality can significantly impact ingestion speed for both Solr and Rocana Search. Our tests include both fixed- and variable-sized data, and the results follow our predicted pattern: Rocana Search’s ingestion rate remains relatively steady while Solr’s decreases over time, mirroring what we’ve seen in much larger production environments.
Query performance
Rocana Search’s query performance is competitive with Solr and can outperform Solr while data ingestion is taking place. In querying for data with varying time windows (six hours, one day, three days), we see Solr returning queries quickly for the fastest 50 percent of the queries. Beyond this, Solr query latency starts increasing dramatically, likely due to frequent multisecond periods of unresponsiveness during data ingest.
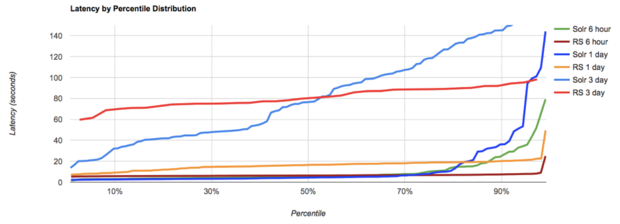
Figure 3: Comparing query latency of Rocana Search versus Solr. Query is for time ranges of six hours, one day, and three days, on a 4.2TB dataset on a four-DataNode Hadoop (HDFS) cluster.
Rocana Search’s behavior under ingest load is markedly different than that of Solr. Rocana Search’s query times are much more consistent, well into the 90th percentile of query times. Above the 50th percentile, Rocana Search’s query times edge out Solr across multiple query range sizes. There are several areas where we anticipate being able to extract additional query performance for Rocana Search as we iterate on the solution, which our customers are already using in production.
A solution for petabyte-scale visibility
Effectively managing today’s complex and distributed business environments requires deep visibility into the applications, systems, and networks that support them. Dissatisfaction with standard approaches led us to develop a unique solution that has already been put into production and been proven to work.
By leveraging the time-ordered nature of operations data and a dynamic sharding model built on Lucene, Rocana Search keeps index sizes reasonable, supports high-speed ingest, and maintains performance by restricting time-oriented searches to a subset of the full data set. As a result, Rocana Search is able to scale indexing and searching in a way that other potential solutions can’t match.
As a group of services coordinated across multiple Hadoop DataNodes, Rocana Search creates shards (partitions and slices) on the fly, without manual intervention, server restarts, or the need to re-index already processed data. Ownership of these shards can be automatically transferred to other Rocana Search nodes when nodes are added or removed from the cluster, requiring no manual intervention.
IT operations data has value. The amount of that data you keep should be dictated by business requirements, not the limitations of your search solution. When enterprises face fewer barriers to scaling data ingest and search, they are able to focus on how to search and analyze as much of their IT operations event data as they wish, for as long as they choose, rather than worrying about what data to collect, what to keep, how long to store it, and how to access in the future.
Brad Cupit and Michael Peterson are platform engineers at Rocana.
New Tech Forum provides a venue to explore and discuss emerging enterprise technology in unprecedented depth and breadth. The selection is subjective, based on our pick of the technologies we believe to be important and of greatest interest to InfoWorld readers. InfoWorld does not accept marketing collateral for publication and reserves the right to edit all contributed content. Send all inquiries to newtechforum@infoworld.com.
Source: InfoWorld Big Data